“Here’s 4 Geppettos for your contested Pinocchios,” Alexandria Ocasio-Cortez tweeted at Washington Post fact-checker Glenn Kessler on January 24. The politician mocked Kessler’s fairy tale-based fact-checking system and scolded him for using a paper by Jason Furman called “Wal-Mart: A progressive success story” in a fact-checking article. Who, Ocasio-Cortez wanted to know, watches the watchmen?
Everybody wants news they can trust, but no one is sure what “trust” means. How would journalists, platforms, or institutions create a concrete theoretical model for credibility, which is a key component of news quality? We would have to provide a framework for distinguishing between different approaches to evaluating credibility and lay the groundwork for a practical approach to measuring credibility at significant scale.
It’s important to note that there is a wide variety of approaches that can be used to evaluate credibility, as well as a variety of organizations and research projects tackling the issue, each through its own particular lens. Some approaches consider content to be less credible if it contains logical fallacies, while others look for underhanded advertising tactics, and others still evaluate whether initial promoters of the content are carrying out some kind of deception, like Twitter accounts that pretend to be grassroots users. Each of these approaches has its merits.
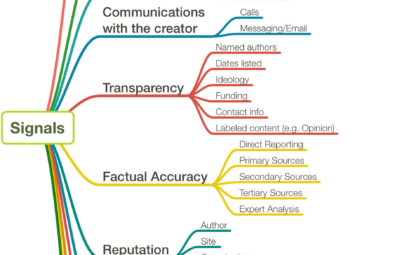
Credibility signals, which affect—and are affected by—the reputation of a news organization, institution, or journalist. Click for full image
What is credibility?
This project’s ultimate goal is to determine what is worthy of trust—to identify the “signals” of the credibility. For this purpose, we define credibility as the likelihood that something informs—or, at least, avoids misleading. Further, we define inform as to improve one’s model of the world—that is, to form accurate beliefs and which can be used to make decisions. Conversely, to mislead is to harm one’s model of the world. So, put holistically, credibility is the likelihood that something enables a person to form accurate beliefs and use them to make effective decisions, especially ones relevant to their lives—or at least avoids decreasing the accuracy of someone’s beliefs or the efficacy of their decisions¹, for example, what medicine to buy or who to vote for.
This definition also has an important caveat: the question of whether or not something actually informs is both interpreter-dependent (dependent on how the consumer of information interprets it) and context-dependent (dependent on the context in which the information is presented). We can’t fully measure the credibility of content or a news source in isolation—we must know about the consumer and their context.²
For our purposes, this definition provides a framework for tackling a key question: “What should we investigate if we want to determine whether something is credible?”
Goals for a credibility assessment system
There are several goals that an ideal credibility assessment system would achieve:
- A method of providing classifications or scores that provide a meaningful measure of credibility as defined above (or the ability to work within a system that will provide a classification or score)
- The trust of as much of the relevant population as possible
- Applicability across platforms and media
- Utility in any language/country
Implicit in the first goal is resilience against being co-opted by authoritarian regimes and other malicious or self-serving actors (as is also true for any reputable news organization).
Credibility Assessment Model
Credibility signals derive from two underlying approaches used to describe the evaluation of credibility—the evidence chain and the reputation network. These approaches are implicitly used by those attempting to assess the credibility of a claim. We call this the Credibility Assessment Model.
The evidence chain frame takes a claim and looks for other claims that either support or contradict it based on observation or analysis. This is the sort of process that might be explicitly carried out by a fact checker to verify a claim. It is an explicit tool, using a chain of links to directly source observation or in-depth analysis and thus assess the credibility of a particular statement. An example of an element in a credibility chain might be “X is true because I saw it” or “X is true according to this economic analysis.”
The reputation network, on the other hand, evaluates claims based on a gestalt of reputation. It’s more similar in function to how an ordinary consumer decides whether to trust an article or a claim they see online: A statement is believed by a consumer if it is from an author the consumer trusts, a website they respect, an institution they believe in, or some combination of the three. The site, author, and institution that each source comes from are not judged independently; they are interdependently linked in a web of trust that determines how credible each individual believes the information to be—the reputation impacts, in other words, go both ways. This is also similar to how PageRank worked to rank Google search results.
The evidence chain is explicit, made of statements like “X’s analysis contains mistakes,” or “X often lies,” while the reputation relationship network is implicit and structural, dependent on relationships like “X is associated with Y” to evaluate credibility.
The evidence chain and the reputation network can also interact. One might factor reputation characteristics in alongside direct evidence when deciding whether or not to trust a claim—researcher X hasn’t made obvious mistakes and has a good reputation, but his work is now hosted by the Y Institute, which has published lies about the topic of his research. When deciding whether or not a specific person, news organization, or institution is trustworthy, one might evaluate them using a separate evidence chain.
And there is at least one further complication—other things affect the reputation network beyond the direct creators. These might include, for example, association with a country that has the ability to influence content for its own geo-political motivations, the use and reputation of a particular ad-tech provider or tag that is found on other sites, or the known influence of a particular twitter botnet on someone who might be acting in good faith. A sophisticated analysis of credibility might incorporate a number influencing entities that can impact the incentives, properties, or capabilities of the creators, including monetizers, nation-states, and distribution platforms.
In summary, the answer to the question “What should we investigate if we want to determine whether something is credible?” is that we need to investigate reputation information, claims with evidence, or some combination of the two.
Credibility Signal properties
With the credibility assessment model in mind, we can now describe a framework for distinguishing between different categories of credibility signal.
Each credibility signal has a subject—the specific aspect of whatever is being evaluated about which it provides information. The signal itself is derived using information from some location(s). That information may be either controlled or not by the creator of the target.
Subject
The subject of a credibility signal refers to what the signal specifically evaluates. This can be the target itself (the content—e.g. the argument and facts stated about Walmart in the paper), the entity who originated the content (the creator—e.g. the paper’s author Jason Furman, or the conference that the paper was written for), or a reference to the content (e.g. the link to the paper in Kenny’s article, which Ocasio-Cortez said tainted it). The content, for example, could be evaluated for logical fallacies. The twitter propagation of the link (a reference) can be evaluated for particular characteristics, and age of the site (a creator) can also be a useful signal.
Location
The location of a signal refers to the place that the evidence or reputation information is collected from. Location directly affects which processes we can use to extract the data; often different environments and platforms make differing types of data available. Evaluating a signal can also sometimes require getting and corroborating data from multiple locations. For example, a system that evaluates whether an article is repeating previously debunked claims requires the evaluator to collect a set of claims that are known to be false (from a directory of fact checkers) and compare that to the article text (found on the creator’s site) to search for those claims.
Control
Control of a signal can either be internal—controlled by the creator—or external—controlled by some other process or entity. For example, if there are logical fallacies in an article, that would be an internal signal, since the creator chooses the content they select for the article. A debunk by a fact checker or even by an anonymous blogger, on the other hand, would be an external signal since it is on a website outside of the creator’s direct control. That said, the lines here are not always fully clear-cut—some information, like website registration dates, can be managed by the creator given enough forethought, but are often not fully in their control—they can’t change the date, or go back in time to choose an earlier date. Control may also be unknown—e.g. it may not be clear if a botnet promoting a story is under the control of the content creator.
There are many potential signals that one can use this framework to interpret; the map below shows just some of the potential credibility signals that might be incorporated in a credibility assessment system in order to make a score.
Credibility measurement in practice
Creation Standards
In the last few years, several standards have emerged to attempt to support the creation of credibility signals. The Claim Review schema, for example, provides a way for third parties to make claims about the accuracy of statement on other sites—it provides a mechanism for an external entity (control), anywhere on the open web (location), to share information about a claim and related (subject). The Trust Project, complementary to that, provides a way for a publisher (subject), to, on their own site (location, control), share reasons and evidence on why they should be trusted. The Credibility Coalition and now the Credible Web Community Group have been exploring the development of standards for third parties (control) to share credibility signals about articles (subject; the author is also affiliated with this effort).
Evaluation Efforts
These standards, and other similar projects, fall into three main categories of evaluation efforts: those focused on helping news consumers directly, those focused on research, and those focused on providing data to platforms and advertisers. Fact checking organizations like those part of International Fact Checking Network provide information to ordinary consumers, based on “manual” human reporting, and generally focusing on the content of a specific claim or article. Source lists like Media Bias Fact Check and Open Sources attempt a similar type of evaluation for news sites.
Research over the past few years has also explored a variety of different signals and sets of signals to evaluate credibility as discussed in the related work section. There has also been a recent profusion of entities focused on providing a credibility score of sorts to third parties, including the Global Disinformation Index and NewsGuard.
Challenges and potential solutions
The ultimate goal of many of these research projects and organizations is to automatically provide meaningful credibility assessments. This would enable any consumer — or platform or advertiser — to easily get an evaluation of whether or not something is credible. However, there are clearly significant challenges with this in practice.
- Human necessity: True fact checking is difficult, human, and expensive—it requires that someone or something follow an evidence chain and discover reputation signals and forms of influence. When the full cost is considered, prominent fact-checking organizations spend hundreds of dollars on a single fact check.
- Insufficient data: Most automated methods require training a machine learning system with many “ground truth” examples, called “training data”, and this can be very expensive to create as it also generally involves human labor.
- Gaming: Once a system can collect signals, and is trained to evaluate those signals—non-credible agents will adapt. This becomes a game of evolving cats-and-mice game.
That said, there are ways to address the problems above and still create assessments that are “good enough.” We can involve a combination of humans and automation working together, with the aim of “significant scale” as opposed to complete coverage. The worst of low-credibility news, which doesn’t even attempt to create a brand or promote a destination, can be largely caught through primarily automated means such as behavioral analysis and use of shared identifiers. Addressing the lowest credibility news is most similar to the battle against spam.
The remaining news can be triaged. This would mean that not everything is checked, but the news receiving the most attention can get meaningful evaluations. A system can collect as much information as possible about the news being evaluated, and, based on that information, feed questions to people with appropriate levels of training or representative knowledge. At the lowest level of training (and for the lowest cost), this is somewhat similar to Mechanical Turk tasks, or Facebook’s survey on trusted news sources. At the upper end, this looks similar to an editor giving an assignment to a trained fact checker—except that this fact checker is given a suite of tools to speed up their research.
A framework for thinking about credibility signals may not give us “the one true answer” on complex cases such whether Glenn Kessler gave AOC an appropriate Pinocchio rating—but it can help us sort through the complexity. Even more importantly, it provides a model for building and understanding systems that combine humans and computers to evaluate credibility at scale—something particularly useful on the open internet, where humans and bots can blend together.
1.This definition evolved from one originally developed to help explore credibility signals in 2016. This version was recently updated based off correspondences with Sandro Hawke around the development of web standards relating to credibility.
2. For simplicity’s sake, in this report we primarily assume a universal interpreter and universal context, but caution that this assumption will often fail in specific circumstances, and is worth ongoing consideration.
An earlier version of this article mischaracterized the relationship between Glenn Kessler and Jason Furman.
Has America ever needed a media defender more than now? Help us by joining CJR today.